The Medical Expenditure Panel Survey
(MEPS) Household Component, a nationally representative sample
of the U.S. civilian noninstitutionalized population, is conducted
by the Agency for Healthcare Research and Quality (AHRQ).
The MEPS Household Component provides national estimates of health
care use, expenditures, sources of payment, and health insurance coverage.
In computing sampling weights for MEPS, base sampling weights are
adjusted for nonresponse and under-coverage in various weighting steps including
a final raking adjustment using control totals for selected demographic
and socio-economic characteristics obtained from the Current Population
Survey (CPS). The MEPS Full Year (FY) weight is released in two steps—a
preliminary weight is released first with the FY Population Characteristics
file, which is revised later for the final FY Consolidated file. The
preliminary weight is produced without using poverty status in the
raking adjustment as the poverty status variable is not available
at that stage. The final weight is produced by adjusting for
poverty status a few months later when the poverty status
variable becomes available along with health care expenditure
variables. This report presents the results from research evaluating
the impact of using education status of family reference person instead
of family poverty status in the raking adjustment. Since education status
is easy to derive and available early, the objective is to see if education status
can be used to improve the preliminary FY weight or if education status can
be used as an alternative to poverty status in producing the final
FY weight.
The estimates in this
report are based on the most recent data available at the time the
report was written. However, selected elements of MEPS data may be
revised on the basis of additional analyses, which could
result in slightly different estimates from those shown
here. Please check the MEPS Web site for the most current file releases.
Background
The Medical Expenditure Panel Survey (MEPS)
Background
The Medical Expenditure Panel Survey (MEPS) is conducted to provide nationally representative estimates of health care use, expenditures, sources of payment, and insurance coverage for the U.S. civilian noninstitutionalized population. MEPS is cosponsored by the Agency for Healthcare Research and Quality (AHRQ), formerly the Agency for Health Care Policy and Research, and the National Center for Health Statistics (NCHS).
MEPS comprises three component surveys: the Household Component (HC), the Medical Provider Component (MPC), and the Insurance Component (IC). The HC is the core survey, and it forms the basis for the MPC sample and part of the IC sample. Together these surveys yield comprehensive data that provide national estimates of the level and distribution of health care use and expenditures, support health services research, and can be used to assess health care policy implications.
MEPS is the third in a series of national probability surveys conducted by AHRQ on the financing and use of medical care in the United States. The National Medical Care Expenditure Survey (NMCES) was conducted in 1977, the National Medical Expenditure Survey (NMES) in 1987. Beginning in 1996, MEPS continues this series with design enhancements and efficiencies that provide a more current data resource to capture the changing dynamics of the health care delivery and insurance system.
The design efficiencies incorporated into MEPS are in accordance with the Department of Health and Human Services (DHHS) Survey Integration Plan of June 1995, which focused on consolidating DHHS surveys, achieving cost efficiencies, reducing respondent burden, and enhancing analytical capacities. To accommodate these goals, new MEPS design features include linkage with the National Health Interview Survey (NHIS), from which the sample for the MEPS-HC is drawn, and enhanced longitudinal data collection for core survey components. The MEPS-HC augments NHIS by selecting a sample of NHIS respondents, collecting additional data on their health care expenditures, and linking these data with additional information collected from the respondents’ medical providers, employers, and insurance providers.
Household Component
The MEPS-HC, a nationally representative survey of the U.S. civilian noninstitutionalized population, collects medical expenditure data at both the person and household levels. The HC collects detailed data on demographic characteristics, health conditions, health status, use of medical care services, charges and payments, access to care, satisfaction with care, health insurance coverage, income, and employment.
The HC uses an overlapping panel design in which data are collected through a preliminary contact followed by a series of five rounds of interviews over a two and a half year period. Using computer-assisted personal interviewing (CAPI) technology, data on medical expenditures and use for two calendar years are collected from each household. This series of data collection rounds is launched each subsequent year on a new sample of households to provide overlapping panels of survey data and, when combined with other ongoing panels, will provide continuous and current estimates of health care expenditures.
The sampling frame for the MEPS-HC is drawn from respondents to NHIS, conducted by NCHS. NHIS provides a nationally representative sample of the U.S. civilian noninstitutionalized population, with oversampling of Hispanics and blacks.
Medical Provider Component
The MEPS-MPC supplements and validates information on medical care events reported in the MEPS-HC by contacting medical providers and pharmacies identified by house-hold respondents. The MPC sample includes all hospitals, hospital physicians, home health agencies, and pharmacies reported in the HC. Also included in the MPC are all office-based physicians:
§
Providing care for HC respondents receiving Medicaid.
§
Associated with a 75 percent sample of households receiving care through an HMO (health maintenance organization) or managed care plan.
§
Associated with a 25 percent sample of the remaining households. Data are collected on medical and financial characteristics of medical and pharmacy events reported by HC respondents, including:
§
Diagnoses coded according to ICD-9 (9th Revision, International Classification of Diseases) and DSMIV (Fourth Edition, Diagnostic and Statistical Manual of Mental Disorders).
§
Physician procedure codes classified by CPT-4 (Current Procedural Terminology, Version 4).
§
Inpatient stay codes classified by DRG (diagnosis related group).
§
Prescriptions coded by national drug code (NDC), medication names, strength, and quantity dispensed.
§
Charges, payments, and the reasons for any difference between charges and payments.
The MPC is conducted through telephone interviews and mailed survey materials.
Insurance Component
The MEPS-IC collects data on health insurance plans obtained through private and public sector employers. Data obtained in the IC include the number and types of private insurance plans offered, benefits associated with these plans, premiums, contributions by employers and employees, and employer characteristics.
Establishments participating in the MEPS-IC are selected through two sampling frames:
§
A Bureau of the Census list frame of private-sector business establishments.
§
The Census of Governments from the Bureau of the Census.
Data from the two sampling frames are collected to provide annual national and State estimates of the supply of private health insurance available to American workers and to evaluate policy issues pertaining to health insurance. Since 2000, the Bureau of Economic Analysis has used national estimates of employer contributions to group health insurance from the MEPS-IC in the computation of Gross Domestic Product (GDP).
The MEPS-IC is an annual panel survey. Data are collected from the selected organizations through a prescreening telephone interview, a mailed questionnaire, and a telephone follow-up for nonrespondents.
Survey Management
MEPS-HC and MPC data are collected under the authority of the Public Health Service Act. Data are collected under contract with Westat. Data sets and summary statistics are edited and published in accordance with the confidentiality provisions of the Public Health Service Act and the Privacy Act. The National Center for Health Statistics (NCHS) of the Centers for Disease Control and Prevention provides consultation and technical assistance related to the selection of the MEPS household sample.
As soon as data collection and editing are completed, the MEPS survey data are released to the public in staged releases of summary reports, micro data files, and tables via the MEPS Web site: www.meps.ahrq.gov. Selected data can be analyzed through MEPSnet, an online interactive tool designed to give data users the capability to statistically analyze MEPS data in a menu-driven environment.
Additional information on MEPS is available from the MEPS project manager or the MEPS public use data manager at the Center for Financing, Access, and Cost Trends, Agency for Healthcare Research and Quality, 540 Gaither Road, Rockville, MD 20850;
301-427-1406, or email MEPSProjectDirector@ahrq.hhs.gov.
AHRQ Publications Clearinghouse
Attn: (publication number)
P.O. Box 8547 Silver Spring, MD 20907
800-358-9295
703-437-2078 (callers outside the United States only)
888-586-6340 (toll-free TDD service;
hearing impaired only)
To order online, send an email to: ahrqpubs@ahrq.gov.
Be sure to specify the AHRQ number of the document or CD-ROM you are requesting. Selected electronic files are available through the Internet on the MEPS Web site: http://www.meps.ahrq.gov/
For more information, visit the MEPS Web site or email mepspd@ahrq.gov.
Return to Table of Contents
1.0 Introduction
The Medical Expenditure Panel Survey (MEPS), conducted by the Agency
for Healthcare Research and Quality (AHRQ), provides nationally representative
estimates of health care use, expenditures, sources of payment, and
health insurance coverage for the U.S. civilian noninstitutionalized
population. It consists of three survey components with the Household
Component (HC) as the core survey. The MEPS Household Component (will
be generally referred to as MEPS hereafter) also provides estimates
of respondents' health status, demographic and socio-economic characteristics,
employment, access to care, and satisfaction with health care. The
MEPS is a complex national area probability sample survey. The sample
for MEPS is selected from the National Health Interview Survey (NHIS)
and contains the same design features as the NHIS. The details of
the NHIS sample design can be found in Botman et al. (2000).
A new panel is sampled for MEPS every year from the previous year’s
responding households of the NHIS. A panel remains in the sample for
two years, which is covered by five rounds of data collection. The
first two interviews (Rounds 1-2) cover most of the first year, the
last two interviews (Rounds 4-5) cover most of the second year, and
the middle interview (Round 3) covers the end part of the first year
and the beginning part of the second year. A MEPS annual file consists
of two overlapping sample panels—the first year of a new panel
and the second year of an old panel. Figure 1 shows an example of the
overlapping of MEPS panels and data collection rounds. The survey can
be used to produce estimates for persons and families as well as subgroups
of the population. The details of the MEPS-HC sample design can be
found in Ezzati-Rice et al. (2008).
Return to Table of Contents
Figure 1. Overlapping of MEPS panels and data collection rounds
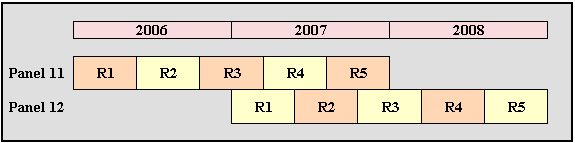
Annual Full Year (FY) files are released in two phases—the preliminary
file (i.e., the FY Population Characteristics file) is released in
the spring and the final file (i.e., the FY Consolidated file) is released
about six months later. Two of the major types of MEPS estimates are
use of health care services and medical expenditures. Since the processing
and derivation of expenditure variables requires more time than that
of population characteristics and utilization variables, a preliminary
file is released earlier so that users can produce estimates related
to population characteristics and health care utilization while waiting
for the expenditure data. Later, when the expenditure variables become
available, the preliminary file is replaced by the final file that
includes use, expenditure, and income data.
The MEPS FY weights are not identical in the preliminary and final
FY files. The preliminary weight released with the preliminary file
is replaced by the final weight when the final file is released.
A series of weighting adjustments for nonresponse, under-coverage,
and
benchmarking to available control totals is applied to produce the
weights for the MEPS FY files. The adjustments are applied separately
for each panel at the end of Round 1, at the end of the first year,
at the end of Round 3, and finally at the end of the second year.
For a FY file, the adjusted weight at the end of the first year
of the
new panel and the adjusted weight at the end of the second year for
the preceding panel are used. When the two panels are combined to
form a FY file, the weights of each panel are scaled down by using
an appropriate
compositing factor to jointly represent the target population. A
raking adjustment is then applied to the composite weight using
a set of raking
dimensions for which the control totals are obtained from the Current
Population Survey (CPS). The variables used in forming the set of
raking dimensions are: age category, sex, race/ethnicity, census
region, metropolitan
statistical area (MSA), and poverty status. The weight released with
the preliminary file is adjusted for all available variables except
for poverty status, which is not available for another four to six
months because it takes longer to derive.
The release of the preliminary file and weight allows users to produce
and analyze some estimates early. However, a disadvantage is that when
the final weight becomes available, if the estimates are not revised,
there may be confusion at a later date about different estimates and
results for the same year. So, it would be convenient to produce the
weight in a single step without any revision. This means either the
weight must be produced in a single step as a final weight when the
poverty variable is available without producing any preliminary weight,
or the preliminary weight must be improved to avoid the need for the
revision. This research evaluates the impact of the poverty status
adjustment on MEPS estimates and investigates if education status of
family reference person can be used to improve the preliminary FY weight
or as an alternative to poverty status in producing the final FY weight.
Since the education variable is derived early, the final weight can
be computed and released early. Another advantage of using education
is that it is easier to collect and derive in a consistent manner with
the source of control totals (i.e., CPS). The derivation of poverty
status is a complex procedure that involves more editing and imputation
that makes it harder to produce poverty status consistent with the
CPS. Such inconsistency in the survey variable and the corresponding
variable in the control total file can introduce noise to the adjustment
process.
Weighting of MEPS FY File
Each MEPS panel is weighted separately for different rounds of nonresponse
and coverage adjustments until the final step, when the two panels
are combined and a raking adjustment is applied to the combined panels
to produce the final FY weight. Figure 2 provides a flowchart of the
weighting scheme used to produce the MEPS FY weights. Machlin, Chowdhury,
et al. (2010) provides details of the weighting and estimation procedures
used in the MEPS.
The weighting of the most recent panel starts with computing the
dwelling unit (DU) base weight, which is calculated by starting
with the nonresponse
adjusted NHIS household weight. A poststratified ratio adjustment
is then applied to the DU base weight to ensure representativeness
of
the MEPS sample in terms of the full NHIS sample. The control total
for this adjustment is derived from the household reference person’s
weight in the NHIS sample. A nonresponse adjustment is applied to the
poststratified DU weight to compensate for the DU nonresponse to the
Round 1 interview. A family-level weight is derived by assigning the
DU weight to each family within the DU and then a family-level poststratification
adjustment is applied using control totals from the CPS. The Round
1 person weight is then derived by assigning the poststratified family
weight to each person in the family and then applying a person-level
poststratification adjustment.
Return to Table of Contents
Figure 2. MEPS Full Year Weighting Scheme
The year 1 person weight is derived by first applying a nonresponse
adjustment to the Round 1 weight for person-level nonresponse up to
the end of the first year (i.e., over Round 2 and year 1 portion of
Round 3) and then applying a raking/poststratification adjustment using
the control total for December 31 of the year derived from the subsequent
March CPS. This produces the year 1 person weight for responding persons
in the most recent panel.
The year 2 weight for the persons in the preceding panel is derived
by starting with the year 1 weight from the previous year and applying
a nonresponse adjustment to compensate for nonresponse in year 2.
A raking/poststratification adjustment is then applied to the nonresponse
adjusted weight. This produces the year 2 weight for responding persons
in the preceding panel.
The two panels are then put together to create the FY file for the
current year. The panel specific annual weights are scaled down
by applying a compositing factor proportional to the sample size
in
each panel so that the composite weights of both panels jointly
adds up
to the size of the target population. Then another round of raking
adjustment is applied to produce the preliminary FY weight for
the cases in the combined panels using the same set of control
totals
used for raking of individual panels. As mentioned in the introduction,
poverty status is not used in the raking adjustment for producing
the
preliminary FY weight. About four to six months later, when the
poverty status becomes available, the raking adjustment is repeated
by adding
dimensions involving poverty status, in addition to the dimensions
used for producing the preliminary FY weights. This produces the
final person-level FY weight. This paper concentrates on these
preliminary and final raking adjustments to assess the impact of
poverty adjustment
and the possibility of replacing the poverty status by education
status
in the raking adjustment.
The Final Raking Adjustment
The variables with their categories used in the final raking adjustment
are: Census region (Northeast, Midwest, South, West), MSA/non-MSA,
race/ethnicity (Hispanic, non-Hispanic black, Asian, others), sex (male/female),
age category (<1 yr, 1-19 yr, 20-29 yr, 45-64 yr, 65+), and poverty
status of the family (below poverty, 100-124 percent, 125-199 percent,
200-399 percent, 400+ percent). A total of 15 raking dimensions are
used for preliminary FY weighting and an additional eight dimensions
involving poverty status are included for the final FY weighting. For
some raking dimensions, the 5-category age group is collapsed to 4-category
or 3-category groups by collapsing some age categories. Table 1 presents
the raking dimensions used for the preliminary and the final raking
adjustments. For the current investigation, another weight (called
education-adjusted weight) is produced by replacing poverty status
with education status of the family reference person to assess the
relative impact of adjustments by poverty and education. The four categories
of education status used are: <12th grade, high school graduate
or GED, some college or associate/vocational degree, and college graduate
or higher.
Return to Table of Contents
Table 1. Raking dimensions used in producing preliminary and final
FY weights
Raking dimensions used in both preliminary & final
weighting
|
Raking dimensions used in final weighting
only
|
1. (Asian/Non-Asian)*Region
|
16. Poverty Status5$
|
2. (Asian, Non-Asian)*(Region West, Other)*
AgeCat4$
|
17. Poverty Status3$*Region*Sex
|
3. (Non-Hispanic Black, Others)*(Region
South, All other)* AgeCat5$
|
18. Poverty Status3*Region*MSA Status
|
4. (Race/Ethnicity3$)*MSA
Status
|
19. Poverty Status3*Sex*AgeCat5
|
5. (Non-Hispanic Black, Others)*(South
Non-MSA, Other)*Sex
|
20. Poverty Status3*Race/Ethnicity3*Sex
|
6. (Hispanic, Others)*(South Non-MSA,
West Non-MSA, Other)*
Sex
|
21. Poverty Status3*Newborn
|
7. Region*Sex* Race/Ethnicity3$
|
22. Poverty Status3*Race/Ethnicity
|
8. Region*Sex* AgeCat5
|
23. Poverty Status3*Region*MSA Status
|
9. Region* Race/Ethnicity3* AgeCat5
|
|
10. Region*MSA Status
|
|
11. (Newborn, Others)*Race/Ethnicity4
|
|
12. (Newborn, Others)*Sex
|
|
13. Race/Ethnicity2*Region*AgeCat5
|
|
14. MSA Status*Sex
|
|
15. MSA Status*AgeCat5
|
|
$AgeCat4=<20, 20-29, 30-44,
45+years; AgeCat5=<20, 20-29, 30-44, 45-64, 65+; race/ethnicity4=Hispanic,
non-Hispanic black, Asian, other; race/ethnicity3=Hispanic, non-Hispanic
black, other; Poverty Status5=below poverty, 100-124 percent,
125-199 percent, 200-399 percent, 400+ percent; Poverty Status3
= below poverty, 100-199 percent, 200+ percent |
Return to Table of Contents
Methodology
To assess the importance of poverty and education in the raking adjustment,
using 2007 FY data, some exploratory analyses are conducted first and
then selected estimates of insurance coverage rates and of utilization
of health care and expenditures under different weighting schemes are
compared.
Under exploratory analyses, the correlation between education and
poverty categories is analyzed first to get an idea of how powerful
education is in explaining poverty status. A model-based analysis is
then conducted of the variances of two important target variables—health
insurance status and total medical expense. A logistic regression is
fitted for insurance status and a general linear model (GLM) is fitted
for total expense with all variables available for raking adjustment
used as independent variables in both cases. Since this is an exploratory
analysis, only main effects of different variables are considered in
the analysis in order to get a broad idea of the relative importance
of different variables.
As part of the exploratory analyses, a preliminary assessment is also
made of the relative impact of adjustment by poverty and/or education
by examining the discrepancy between estimated population totals and
the known control totals and variation in insurance coverage rates
in different poverty or education categories. This gives an indication
of the direction and extent of an impact on insurance coverage if an
overall adjustment is made by poverty only, by education only, or by
education in addition to poverty.
The final assessment is made by comparing important MEPS estimates
such as the estimates of insurance coverage and selected utilization
and expense estimates under three raking adjusted weights. The three
raked weights used in producing the estimates are as follows:
- Preliminary FY weight - produced with raking adjustment
without including poverty or education in the raking dimensions.
- Final poverty-adjusted FY weight - produced by including
all raking dimensions used in producing the preliminary weight
plus the
dimensions involving poverty status.
- Education-adjusted FY weight - produced by including
all raking dimensions used in preliminary weighting plus the dimensions
formed
by replacing poverty status with education status in the raking
dimensions used for producing the final FY weight.
The first two weights are already available in the 2007 MEPS preliminary
and final FY files and did not require calculation. However, the education-adjusted
weight was calculated by using a raking algorithm that used the preliminary
FY weight as the starting weight and the raking dimensions included
all dimensions used in producing preliminary weights plus the dimensions
involving education instead of poverty. Another weight by simultaneously
including both poverty and education in the raking adjustment was considered
but was not produced due to the problem with convergence of the raking
algorithm with too many dimensions. However, a simple analysis that
does not require computing such raked weight is presented as part of
the exploratory analysis to assess the potential impact of such an
adjustment. Considering the poverty-adjusted weight as the current
standard, the estimates without adjustment by education or poverty
(i.e., preliminary estimates) and the estimates based on the education
adjustment (alternative estimates) are compared with the estimates
under the final weight (i.e., final estimates). The comparison of preliminary
and final estimates shows the impact of poverty status adjustment,
and the comparison of alternative estimates and final estimates shows
the effectiveness of the education adjustment as an alternative to
the poverty status adjustment.
If the preliminary or the alternative estimate is considered biased
compared to the final estimate, then the difference between estimates
can be considered as an estimate of bias. To assess if this bias
can be ignored in practice, in addition to the statistical significance,
we also analyzed the practical significance of the difference. Because
the hypothesis of ‘a bias not equal to zero’ may be statistically
significant, but the bias may not be large compared to the SE of
the biased estimate to have much impact on the inference, i.e., the
confidence interval coverage for the target parameter. Therefore,
in assessing the practical importance of a difference (diff) between
a biased (preliminary or alternative) estimate with the unbiased
(final) estimate, the difference is related to the standard error
(SE) of the biased estimate. The ratio of the difference over the
SE of the estimate (diff/SE) shows the extent of difference in a
standardized scale. If a 95 percent confidence interval (CI) is formed
for the population parameter using the biased estimate, then diff/SE
will be comparable to standardized normal values and will give an
indication of the actual performance of the CI in covering the unbiased
estimate.
Figure 3 shows the actual CI coverage under three levels of bias (i.e.,
1.96SE, 1.0SE, and 0.5SE) assuming that the SE is roughly the same
for both biased and unbiased estimators. It shows that when the bias
is 1.96SE the 95 percent CI coverage is only 50 percent instead of
95 percent, if the bias is 1.0SE then the CI coverage is about 83 percent,
and when the bias is 0.5SE the CI coverage is 92.1 percent. Table 2
summarizes the relationship between bias, SE, CI coverage, and mean
square error (MSE) over variance. It shows that CI coverage goes below
90 percent as bias/SE goes above 0.6SE.
Return to Table of Contents
Figure 3. CI coverage under different levels of bias
(The curve in black shows the distribution under the biased estimate,
the curve in red shows the distribution under the unbiased estimate,
and shaded blue area shows the type I error)
Cochran (1977) provides further discussion on this topic and suggests
keeping |bias/SE| below 0.20 to keep the CI coverage as close as possible
to 95 percent. In the case of this analysis, we will consider a bias
up to 0.50SE (i.e., |bias/SE|<0.5) ignorable considering the negligible
practical implications of such a bias.
Table 2. Relationship between bias, SE, CI coverage, and mean square
error over variance
|Bias/SE| |
Type I error |
Actual coverage of 95 percent CI |
Increase in MSE/Var (square of bias/SE) |
0.00 |
0.050 |
0.950 |
0% |
0.20 |
0.055 |
0.945 |
4% |
0.30 |
0.060 |
0.940 |
9% |
0.40 |
0.069 |
0.931 |
16% |
0.50 |
0.079 |
0.921 |
25% |
0.60 |
0.092 |
0.908 |
36% |
0.75 |
0.117 |
0.883 |
56% |
1.00 |
0.170 |
0.830 |
100% |
1.50 |
0.323 |
0.677 |
225% |
1.65 |
0.378 |
0.622 |
272% |
1.96 |
0.500 |
0.500 |
384% |
Return to Table of Contents
Results
Exploratory Analysis
Association between education and poverty
Table 3 presents percentage distributions of persons in poverty categories
within each family-level education category and vice versa, i.e.,
row and column percentages in the cross tabulation of poverty and
education. The two highest percentages in each row and column are
marked (one asterisk on highest row values, two asterisks on highest
column values). For a very strong association between education and
poverty, the highlighted values should be high and concentrated along
the diagonal. Here, the highlighted values are moderately concentrated
along the diagonal indicating a moderate level of association between
education status and poverty status. The last row in the table shows
that the Pearson correlation between the categories of the two variables
is 0.42.
Table 3. Association between education and poverty—percentage
distributions of poverty categories within each education category
and vice versa
Poverty status=>
Education status
|
Poor |
Near poor |
Low income |
Middle income |
High income |
Total row percentage |
12th grade or less |
32.98* |
18.93 |
14.38 |
25.54* |
8.13 |
100.0 |
35.39** |
29.22** |
22.70 |
12.24 |
3.47 |
|
HS graduate/GED |
16.27 |
11.92 |
11.62 |
36.36* |
23.83* |
100.0 |
38.06** |
40.11** |
39.97** |
37.96** |
22.05 |
|
Some college, associate or vocational degree |
11.20 |
8.47 |
9.95 |
34.97* |
35.41* |
100.0 |
19.11 |
20. 77 |
24.96** |
26.63** |
23.90** |
|
College degree and above |
3.68 |
3.40 |
4.15 |
25.63* |
63.14* |
100.0 |
7.44 |
9.89 |
12.37 |
23.17 |
50.58** |
|
Total column percentage |
- |
- |
- |
- |
- |
|
100.0 |
100.0 |
100.0 |
100.0 |
100.0 |
|
Pearson correlation=0.42 (p<.005) |
Return to Table of Contents
Analysis of variance of target variables
Tables 4 and 5 present analysis of variance of two important MEPS target
variables in terms of all base raking variables plus poverty and
education statuses.
Table 4 presents an analysis of variance from a logistic regression
model of health insurance status (no/yes). It shows that after
including all base variables in the model, both poverty and education
are still
significant to explain further variation in insurance status. However,
the values of chi-square indicate that poverty is considerably
more powerful than education in explaining the variation in insurance
status
(even if the difference in degrees of freedom is taken into account).
Overall, poverty status appears to be the second most important
variable (after age) in explaining the variation in insurance status.
Table 4. Type III analysis of variance for insurance status
Source |
Degrees of freedom |
Chi-square |
Pr>Chi-square |
Age category |
4 |
1280.9 |
<.0001 |
Newborn |
1 |
0.1 |
0.9422 |
Sex |
1 |
87.0 |
<.0001 |
Race/ethnicity |
3 |
206.1 |
<.0001 |
Region |
3 |
159.3 |
<.0001 |
MSA |
1 |
4.2 |
0.0395 |
Poverty status |
4 |
710.4 |
<.0001 |
Education status |
3 |
188.4 |
<.0001 |
Return to Table of Contents
Table 5 presents a similar analysis of variance from a general linear
model of total health care expense. Poverty and education are both
significant in explaining the variation in total expense but the values
of chi-square show that these two variables are not as important for
explaining variation in total expense as in the case of insurance status.
Table 5. Type III analysis of variance for total health care expense
Source |
Degrees of freedom
|
Chi-square
|
Pr>Chi-square
|
Age category
|
4
|
387.3 |
<.0001 |
Newborn
|
1
|
7.4 |
0.0065 |
Sex
|
1
|
15.7 |
<.0001 |
Race/ethnicity
|
3
|
13.5 |
<.0001 |
Region
|
3
|
2.6 |
0.0492 |
MSA
|
1
|
0.3 |
0.6178 |
Poverty status
|
4
|
7.5 |
<.0001 |
Education status
|
3
|
4.0 |
<.0070 |
Preliminary assessment of impacts of adjustments
A preliminary assessment of the impact of adjustments by poverty versus
education is also made using a simple approach by comparing the variation
of the insurance coverage rates and adjustment factors across the categories
of poverty and education. Table 6 shows that the estimates of percentage
not-insured (using the preliminary weight) vary considerably by the
categories of poverty status. The third and fourth columns of the table
present the estimated (using the preliminary weight) and the actual
(from the CPS) population totals. The adjustment factors (i.e., the
ratios of known population control totals over the estimated population
totals before adjustment) also vary considerably. The population with
high income is underrepresented in the sample while their insurance
coverage is higher. In fact, there is a strong negative association
between percentage not-insured and the adjustment factors, implying
that the adjustment by poverty would reduce the overall estimate of
percentage not-insured. The last row of the table shows that a simple
adjustment by poverty status reduces the overall estimate of percentage
not-insured from 18.22 percent to 17.62 percent. These overall estimates
of percentage not-insured are obtained by weighting the percentage
not-insured in each category by the estimated and actual population
totals in each category, respectively.
Return to Table of Contents
Table 6. Assessing the impact of adjustment by poverty status
Family poverty status |
(2)
Persons not insured1
|
(3)
Total population before adjustment1
|
(4)
Control total
|
(5)
Ratio= (4)/(3)
|
Correlation between
(2 & 5)
|
Poor |
28.86% |
42,188,956 |
38,157,957 |
0.9045 |
-0.99 |
Near poor |
28.30% |
29,329,645 |
27,299,939 |
0.9308 |
|
Low income |
27.81% |
28,677,420 |
26,472,483 |
0.9231 |
|
Mid income |
18.81% |
94,486,629 |
93,233,728 |
0.9867 |
|
High income |
8.13% |
106,626,499 |
116,145,042 |
1.0893 |
|
Total |
|
301,309,149 |
301,309,149 |
|
|
Overall not insured |
|
18.22% |
17.62% |
|
|
1Estimated using preliminary weights |
Table 7 presents a similar analysis for an adjustment by education
category. The last row of the table shows that a simple adjustment
by education status reduces the overall estimate of percentage not-insured
from 18.22 percent to 17.97 percent. Both adjustments by poverty and
education would have a downward impact on the estimate of percentage
not-insured but the impact of adjustment by poverty is greater than
the impact of adjustment by education. Since this approach does not
require computing the raked weight including poverty and education,
table 8 presents a similar analysis to show the likely impact of adjustment
by education on top of the adjustment by poverty. The correlation between
percentages not-insured and adjustment factors by education categories
is very low (after adjustment for poverty status), which is reflected
in the small change in the overall estimate (17.62 versus 17.55).
Return to Table of Contents
Table 7. Assessing the impact of adjustment by education status
Education status of family reference person
|
(2)
Persons not insured1
|
(3)
Total population before adjustment1
|
(4)
Control total
|
(5)
Ratio= (4)/(3)
|
Correlation between
(2 & 5)
|
12th grade or less
|
30.11% |
45,271,845 |
43,244,700 |
0.9552 |
-0.42 |
HS graduate/GED
|
21.00% |
98,664,521 |
88,807,243 |
0.9001 |
|
Some college
|
17.19% |
71,963,494 |
82,321,913 |
1.1439 |
|
College grad + |
9.56% |
85,409,289 |
86,935,293 |
1.0179 |
|
Total
|
|
301,309,149 |
301,309,149 |
|
|
Overall not insured
|
|
18.22% |
17.97% |
|
|
1Estimated using preliminary
weights |
Return to Table of Contents
Table 8. Assessing the impact of adjustment by education status in
addition to poverty status
Education status of family reference person
|
(2)
Persons not insured1
|
(3)
Total population before adjustment1
|
(4)
Control total
|
(5)
Ratio= (4)/(3)
|
Correlation between
(2 & 5)
|
12th grade or less
|
29.80% |
43,617,811 |
43,244,700 |
0.9914 |
-0.14 |
HS graduate/GED
|
20.52% |
96,693,329 |
88,807,243 |
0.9184 |
|
Some college
|
16.60% |
72,066,328 |
82,321,913 |
1.1423 |
|
College grad + |
9.32% |
88,931,681 |
86,935,293 |
0.9776 |
|
Total
|
|
301,309,149 |
301,309,149 |
|
|
Overall not insured
|
|
17.62% |
17.55% |
|
|
1Estimated using poverty adjusted
weights |
Return to Table of Contents
Comparison of Estimates
Tables 9 and 10 present a comparison of estimates under the three
adjustment schemes. The differences of the estimates with the final
poverty-adjusted estimates, SEs, and corresponding ratios of difference
over SE (diff/SE) are presented. Differences in SEs of estimates under
different adjustments are mostly very small, which is consistent with
the coefficient of variations (CVs) of different weights. The CVs of
the three weights are 71.1 percent for the preliminary weight and 73.2
percent for both education-adjusted and final weights. So we will mainly
concentrate on the difference (bias) in estimates. As mentioned in
the methodology section, in addition to statistical significance of
the difference, implications of the difference on the confidence intervals
will be considered. The differences significant at 0.05 or lower level
are indicated by an asterisk. However, as discussed before, any |diff/SE|
less than 0.50 will be considered ignorable even if the difference
is statistically significant.
Table 9 presents the percentage distribution across different
insurance categories by age, race/ethnicity, and MSA status under different
weighting adjustments. Most preliminary and education-adjusted
estimates of percentages
in insurance categories are significantly different than the final
estimates. As noted in the table (marked with a dagger symbol),
most of the significant differences are non-ignorable as |diff/SE|
is greater than 0.50. For example,
the overall estimate of private insurance coverage is 61.90 percent
under
the final weight, 60.32 percent (1.58 percentage points lower) under
the preliminary weight and 60.91 percent (0.99 points lower) under
the education adjusted weight. The differences are statistically
significant (as indicated by asterisks) and |diff/SE| is substantially
higher than
0.50 in both cases. Similarly, the estimate of never insured is 17.76
percent under the final weight, 18.36 percent under the preliminary
weight, and 18.07 percent under the education-adjusted weight. The
differences between estimates are significant and corresponding |diff/SE|
in both cases are considerably greater than 0.50. The table presents
similar comparisons by selected categories of age, race/ethnicity,
and by MSA status. The pattern of differences is similar and in most
cases the differences are both significant and non-ignorable. However,
the differences between the education-adjusted and the final estimates
are smaller (by 30 percent to 50 percent in most cases) than the
differences between the preliminary and the final estimates. So,
the comparison
of estimates in table 9 shows that, for insurance coverage estimates,
adjustment by poverty status is non-ignorable and the adjustment
by education status makes some improvement from the preliminary
estimates
but is not as effective as the adjustment by poverty status.
Table 10 presents similar comparisons for selected use and expenditure
variables overall and by major age categories. In contrast to insurance
coverage, the differences between preliminary or education-adjusted
estimates and the final estimates are mostly not significant and
ignorable as |diff/SE| is less than 0.50 in the majority of cases.
For example,
for mean overall health care expenditures, the final estimate is
$4,404, the preliminary estimate is $4,399, and the education adjusted
estimate
is $4,399.7. These differences are not significant and also ignorable.
The pattern is the same for most of the other expenditures and
use estimates overall and for subpopulations, except for the mean
number
of dental visits, where the differences between the preliminary
and the final estimates are not ignorable in all cases. In some
cases,
although the difference is significant, it can be ignored because
|diff/SE| is less than 0.50.
Return to Table of Contents
Table 9. Comparison of preliminary and education-adjusted estimates
with final (poverty-adjusted) estimates for insurance coverage status
|
Final estimate (poverty adjusted)
| Preliminary estimate (no
poverty or education adjustment)
| Alternative estimate (education-adjusted)
|
Insurance status on 12/31/07
|
Estimate (SE0)
| Estimate (SE1)
| diff1
| diff/ SE1
| Estimate (SE2)
| diff1
| diff/ SE2
|
All
|
Private
|
61.90 (0.62)
| 60.32 (0.63)
| -1.58*†
| -2.51†
| 60.91 (0.63)
| -0.99*†
| -1.57†
|
Public only
|
20.35 (0.42)
| 21.32 (0.45)
| 0.97*†
| 2.15
†
| 21.02 (0.45)
| 0.67*
†
| 1.49†
|
Never insured
|
17.76 (0.42)
| 18.36 (0.43)
| 0.60*†
| 1.39
†
| 18.07 (0.43)
| 0.31*†
| 0.72†
|
Under 18 years
|
Private
|
55.46 (1.11)
| 52.73 (1.13)
| -2.73*†
| -2.42†
| 53.59 (1.13)
| -1.87*†
| -1.65†
|
Public only
|
32.17 (0.97)
| 34.65 (1.02)
| 2.48*†
| 2.43†
| 33.89 (1.02)
| 1.72*†
| 1.69†
|
Never insured
|
12.37 (0.67)
| 12.62 (0.67)
| 0.25*
| 0.37
| 12.51 (0.68)
| 0.14
| 0.21
|
18-64 years
|
Private
|
67.22 (0.59)
| 65.80 (0.60)
| -1.42*†
| -2.37†
| 66.46 (0.59)
| -0.76*†
| -1.29†
|
Public only
|
9.53 (0.32)
| 10.08 (0.34)
| 0.55*†
| 1.62†
| 9.84 (0.34)
| 0.31*†
| 0.91†
|
Never insured
|
23.26 (0.51)
| 24.12 (0.51)
| 0.86*†
| 1.69†
| 23.70 (0.51)
| 0.44*†
| 0.86†
|
Hispanics
|
Private
|
37.34 (1.26)
| 35.89 (1.25)
| -1.45*†
| -1.16†
| 36.80 (1.28)
| -0.54*
| -0.42
|
Public only
|
28.64 (0.95)
| 29.46 (0.95)
| 0.82*†
| 0.86†
| 29.09 (0.96)
| 0.45*
| 0.47
|
Never insured
|
34.03 (1.09)
| 34.65 (1.09)
| 0.62*†
| 0.57†
| 34.11 (1.09)
| 0.08
| 0.07
|
Non-Hispanic blacks
|
Private
|
48.15 (1.29)
| 45.70 (1.27)
| -2.45*†
| -1.93†
| 46.66 (1.30)
| -1.49*†
| -1.15†
|
Public only
|
31.92 (1.18)
| 33.70 (1.19)
| 1.78*†
| 1.5†
| 33.05 (1.21)
| 1.13*†
| 0.93†
|
Never insured
|
19.93 (0.83)
| 20.60 (0.85)
| 0.67*†
| 0.78†
| 20.29 (0.86)
| 0.36
| 0.42
|
MSA
|
Private
|
62.59 (0.67)
| 61.12 (0.68)
| -1.47*†
| -2.16†
| 61.82 (0.68)
| -0.77*†
| -1.13†
|
Public only
|
19.68 (0.44)
| 20.51 (0.47)
| 0.83*†
| 1.77†
| 20.17 (0.46)
| 0.49*†
| 1.07†
|
Never insured
|
17.74 (0.48)
| 18.28 (0.48)
| 0.54*†
| 1.25†
| 18.00 (0.48)
| 0.26*†
| 0.54†
|
Non-MSA
|
Private
|
58.28 (1.57)
| 55.68 (1.61)
| -2.6*†
| -1.61†
| 56.16 (1.65)
| -2.12*†
| -1.28†
|
Public only
|
23.85 (1.24)
| 25.56 (1.30)
| 1.71*†
| 1.32†
| 25.46 (1.35)
| 1.61*†
| 1.19†
|
Never insured
|
17.87 (0.82)
| 18.76 (0.86)
| 0.89*†
| 1.03†
| 18.39 (0.85)
| 0.52
| 0.61
|
1Difference
from the final estimate, *indicates significant at 5 percent
or below level, †indicates |diff/SE| is greater than
0.50 |
Return to Table of Contents
Table 10. Comparison of preliminary and education-adjusted estimates
with final (poverty-adjusted) estimates for selected use and expenditure
variables
|
Final
estimate (poverty adjusted)
|
Preliminary
estimate (no poverty or education adjustment)
|
Alternative
estimate (education-adjusted)
|
Use and expense variabless |
Estimate (SE0)
|
Estimate
(SE1)
|
diff1
|
diff/ SE1
|
Estimate
(SE2)
|
diff1
|
diff/ SE2
|
Mean # of office visits
|
Overall
|
4.98 (0.08)
|
4.95 (0.08)
|
-0.03*
|
-0.38
|
4.98 (0.08)
|
0.00
|
0.00
|
Under 18
|
2.78 (0.09)
|
2.74 (0.09)
|
-0.04*
|
-0.44
|
2.77 (0.09)
|
-0.01
|
-0.11
|
18-64 yrs
|
4.73 (0.10)
|
4.71 (0.10)
|
-0.02*
|
-0.20
|
4.75 (0.10)
|
0.02
|
0.20
|
65+ Yrs
|
10.36 (0.26)
|
10.31 (0.26)
|
-0.05
|
-0.19
|
10.30 (0.25)
|
-0.06
|
-0.24
|
Mean # of dental care visits
|
Overall
|
1.01 (0.02)
|
0.99 (0.02)
|
-0.02*† |
-1.00†
|
1.00 (0.02)
|
-0.01
|
-0.50
|
Under 18
|
1.10 (0.03)
|
1.06 (0.03)
|
-0.04*†
|
-1.33†
|
1.08 (0.03)
|
-0.02
|
-0.67
|
18-64 yrs
|
0.94 (0.02)
|
0.92 (0.02)
|
-0.02*†
|
-1.09†
|
0.93 (0.02)
|
-0.01
|
-0.50
|
65+ yrs
|
1.20 (0.04)
|
1.19 (0.04)
|
-0.01
|
-0.25
|
1.19 (0.04)
|
-0.01
|
-0.25
|
Mean health care expense2
|
Overall
|
4404.2 (101.30)
|
4399.0 (98.62)
|
-5.2
|
-0.05
|
4399.7 (99.72)
|
-4.5
|
-0.05
|
Under 18
|
1620.1 (131.05)
|
1615.7 (139.15)
|
-4.4
|
-0.03
|
1616.2 (138.70)
|
-3.9
|
-0.03
|
18-64 yrs
|
4265.3 (133.31)
|
4262.4 (126.19)
|
-2.9
|
-0.02
|
4275.1 (130.22)
|
9.8
|
0.08
|
65+ yrs
|
9696.4 (291.10)
|
9655.7 (290.11)
|
-40.7
|
-0.14
|
9628.3 (285.36)
|
-68.1
|
-0.24
|
Mean expense paid by self or family2
|
Overall
|
714.7 (14.24)
|
708.7 (14.11)
|
-6.0*
|
-0.43
|
712.5 (14.27)
|
-2.2
|
-0.15
|
Under 18
|
294.4 (14.87)
|
283.2 (14.09)
|
-11.2*†
|
-0.79†
|
289.8 (14.72)
|
-4.6
|
-0.31
|
18-64 yrs
|
740.7 (14.99)
|
736.3 (14.77)
|
-4.4*
|
-0.30
|
741.6 (15.11)
|
0.9
|
0.06
|
65+ yrs
|
1318.3 (60.3)
|
1311.6 (60.23)
|
-6.7
|
-0.11
|
1307.7 (59.87)
|
-10.6
|
-0.18
|
Mean prescription drug expense2
|
Overall
|
909.9 (21.09)
|
918.1 (21.29)
|
8.2
|
0.38
|
917.2 (21.26)
|
7.3
|
0.34
|
Under 18
|
198.6 (17.32)
|
200.6 (18.29)
|
2.0
|
0.11
|
200.5 (17.58)
|
1.9
|
0.11
|
18-64 yrs
|
889.6 (25.11)
|
899.5 (24.87)
|
9.9
|
0.40
|
899.5 (24.98)
|
9.9
|
0.40
|
65+ yrs
|
2198.9 (71.83)
|
2204.2 (74.48)
|
5.3
|
0.07
|
2204.3 (74.27)
|
5.4
|
0.07
|
Mean dental care expense3
|
Overall
|
642.9 (14.11)
|
640.0 (14.15)
|
-2.9*
|
-0.20
|
641.5 (14.03)
|
-1.4
|
-0.10
|
Under 18
|
565.2 (29.02)
|
558.0 (29.07)
|
-7.2*
|
-0.25
|
560.0 (29.13)
|
-5.2*
|
-0.18
|
18-64 yrs
|
647.4 (17.00)
|
645.0 (17.00)
|
-2.4*
|
-0.14
|
647.2 (16.86)
|
-0.15
|
-0.01
|
65+ yrs |
776.2 (46.75) |
777.7 (48.31) |
1.5 |
0.03 |
776.7 (47.44) |
0.50 |
0.01 |
1Difference from
the final estimate, *indicates significant at 5 percent or below
level, †indicates |diff/SE| is greater than 0.50, 2Who
had total expense>0, 3Who
had dental care expense
|
Return to Table of Contents
Conclusion
The MEPS final FY weights are produced by including dimensions involving
family poverty status in the raking adjustment. Since the derivation
of poverty status involves a complex procedure, it delays the production
of the final FY weights. This study examines the option of using education
status of family reference person instead of family poverty status
in the final raking adjustment.
Analyses of variances of some important MEPS target variables show
that both poverty and education are significant in explaining variation
in insurance status but not as important for use and expenditure
variables. However, poverty status is substantially more effective
than education
status in explaining the variation in insurance status. A correlation
analysis shows that education status is only moderately associated
with poverty status.
A comparison of estimates based on different weights shows that raking
adjustment by poverty status appears to have significant and non-ignorable
impacts for insurance coverage estimates. The differences between
preliminary (not adjusted by poverty or education) and final (adjusted
by poverty
status) estimates are generally significant and non-ignorable. Adjustment
by education status instead of poverty status puts the estimates
closer to the final estimates but the differences with the final
estimates
are still significant and non-ignorable. For most use and expenditure
estimates, differences between preliminary and final estimates are
significant in some cases, but ignorable in most cases, and differences
between education-adjusted and final estimates are both non-significant
and ignorable in most cases.
The estimates based on the weight from the raking adjustment using
both education and poverty is not compared because of the difficulty
with convergence with too many raking dimensions. A weight with
some collapsing or modifications of raking dimensions could still
be produced,
however this was not done as the analysis of variance and a simple
adjustment showed that the impact of adjustment by education in
addition to poverty would be negligible.
The overall analysis suggests that education status can be used
as a replacement for poverty status in raking adjustment for
use and
expenditure estimates, but is not effective enough as a replacement
for poverty
status for the estimates of insurance coverage. However, since
the education adjustment puts the estimates closer to the final
estimates,
one possibility is to add education status in the raking adjustment
to produce the preliminary weight. That will reduce the differences
between preliminary and final weights and estimates.
References
Botman S.L., Moore T.F., Moriarity C.L. (2000). Parsons V.L. Design
and Estimation for the National Health Interview Survey, 1995–2004.
National Center for Health Statistics. Vital Health Stat 2(130).
Cochran W.G. (1977). Sampling Techniques. New York, John Wiley & Sons,
Inc.
Ezzati-Rice, T.M., Rohde, F., Greenblatt, J. (2008). Sample Design
of the Medical Expenditure Panel Survey Household Component, 1998–2007.
Methodology Report No. 22. March 2008. Agency for Healthcare Research
and Quality, Rockville, MD. http://www.meps.ahrq.gov/mepsweb/data_files/publications/mr22/mr22.shtml
Machlin S.R., Chowdhury S.R., Ezzati-Rice T., DiGaetano R., Goksel
H., Wun L.-M., Yu W., Kashihara D. (2010). Estimation Procedures
for the Medical Expenditure Panel Survey Household Component. Methodology
Report #24. September 2010. Agency for Healthcare Research and Quality,
Rockville, MD. http://www.meps.ahrq.gov/mepsweb/data_files/publications/mr24/mr24.shtml
Return
to Table of Contents